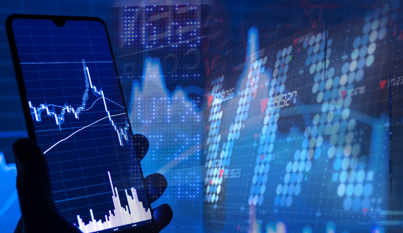
By Shawn Hutchins
Research at Rice University’s Center for Computational Finance and Economic Systems (CoFES) is implementing time-series methods and machine learning to analyze intraday stock volume and forecast seasonal change.
The research is part of a new program made possible by the generous support of Vanguard’s Fintech Ventures team (VFV).
Initiated by Rice statistics alumna Lada Kyj, Ph.D., head of VFV’s Quant Fintech Strategies efforts at Vanguard, the program explores emerging technologies to build new insights and new capabilities that can help Vanguard’s investors succeed well into the future. In addition to the core research efforts, Vanguard is interested in developing emerging and diverse talent for the fintech workforce.
Stock price movements aren't always what they appear. To understand what’s happening, quantitative investors rely on a slate of market volume indicators when looking to confirm or refute a trend or assess a particular strength behind trading intensities.
This past spring, statistics doctoral student Mike Jackson led a project to develop and test a hybrid NARX-based seasonal predictive model called the Seasonal Nonlinear Autoregressive Neural Network with Exogenous Variables (S-NARX).
Also working as research assistants on the project were statistics professional master’s student Hanxuan Lin, Kelly Liu, who is a senior majoring in statistics, and Gazi Fuad, a senior majoring in statistics and mathematical economics. The students conducted literature reviews of previous studies and helped create foundational analysis and data visualizations of the trading volume.
Intraday volume data is known to fluctuate throughout the trading day, sometimes according to common holidays, business cycles, or during economic growth or increases in production over a given time frame.
“The S-NARX model takes advantage of information found in the data up until the auction time and high-frequency intraday trading volume’s cyclical seasonal pattern to predict end-of-day volume,” said Jackson, who defended his thesis the past May with Katherine Ensor, the Noah G. Harding Professor of Statistics and director of CoFES serving as his adviser. Jackson is now a quantitative analyst at Houston-based startup New Territory Advisors, LLC, where he leads the Data Science and Machine Learning Division.
There have been several studies focused on predicting intraday volume, the motivation for this study is unique in the way it combines the use of an autoregressive-moving-average model with machine learning techniques to forecast the minute-by-minute end-of-day trading volume over the course of a year,” said Jackson.
In this momentum-based study, the team specifically focused on the model’s ability to predict the transformation of trading volume over the last 15 minutes of the trading day. Daily seasonal patterns in intraday volume data were well documented.
Since its founding in 2002, CoFES has been dedicated to the advancement of quantitative finance and economic systems and their ultimate impact on society. Using modern computational, statistical and mathematical techniques for modeling complex problems, the center strives to deliver world-class research, expand education and advance public knowledge.